[ad_1]
Diffusion models are a significant component in generative models, particularly for image generation, and these models are undergoing transformative advancements. These models, functioning by transforming noise into structured data, especially images, through a denoising process, have become increasingly important in computer vision…
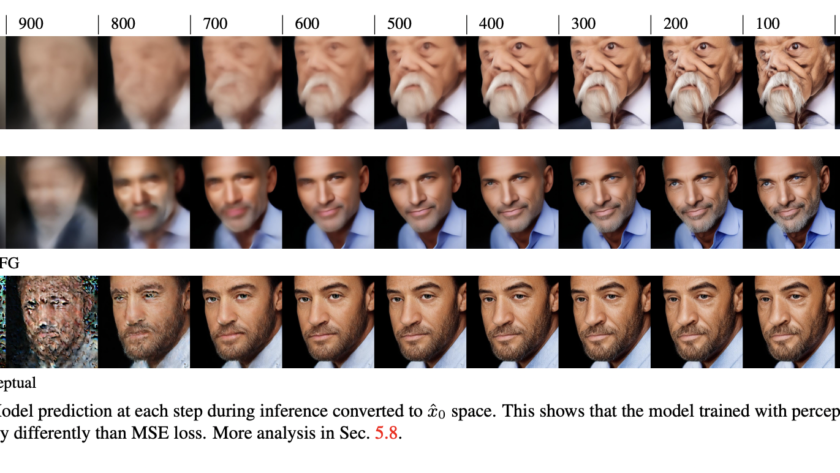