[ad_1]
An illustrated and intuitive guide on how Neural Networks learn Welcome to Part 2 of the Deep Learning Illustrated series. In the previous article (definitely read that first!), we covered how a neural network works and how a trained neural network makes predictions. In this article, we’ll delve into the training process and explore…
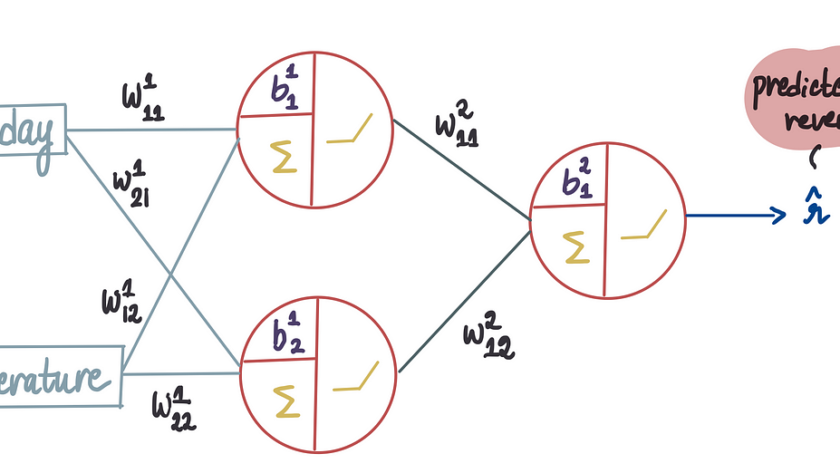